3 Questions to Ask Before Implementing AI
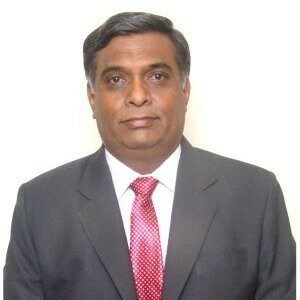
Sudhakar Nagasampagi
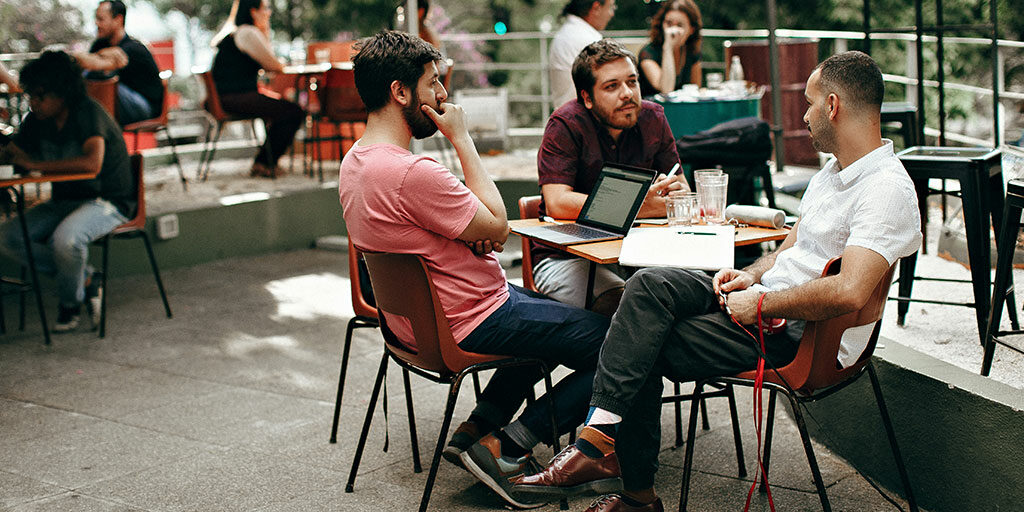
Many organizations ventured into AI with the hope of solving a multitude of different organizational concerns. This, in turn, they fathomed, would considerably improve their profits and market position. Initial results in this race were encouraging, resulting at times in a further investment of millions of dollars.
Several organizations achieved wonders but many others were left devastated when their ventures failed. In the haste to beat the competition and reach the peak, many basic questions that ought to be asked before implementing AI were either overlooked or poorly addressed.
Organizations need to do a full-fledged reality-check before taking up an AI project. It’s absolutely crucial that organizations learn from past mistakes and hire the very best AI professionals they can find. For all the merits and importance of the initiatives I’ll mention, the likeliness of “no” being the answer to at least one of the three questions prior to implementing AI, is high.
Table of Contents
Do We Have an Adequate Vision and Feasible Strategy?
When Apple released iPhone X, its Facial Recognition was presented as one of the key capabilities. However, this very feature failed when it got fooled by a plastic mask that was proven to be able to unlock the phone. Another major flaw detected was its inability to differentiate between identical twins. The ambition of the vision of implementing facial recognition was not met by a feasible strategy.
This is not an isolated case. “Todai Robot”, which was created to crack the entrance exam and gain admission to the University of Tokyo is another example of implemented AI that did not meet its high aspirations. It failed the exam just as humans do. The robot wasn’t smart enough to understand the broader implications of the questions asked. A year later, the robot made another attempt but failed again. Researchers abandoned the project then.
Were the Algorithms Appropriately Tested?
It can happen that organizations do not allocate the required time and resources into ensuring their algorithms are extensively tested. IBM partnered with renowned doctors and cancer centers in using AI for their product Watson to diagnose and treat cancer patients. The product came under severe criticism for providing erroneous and dangerous treatment recommendations. If there’s one field where we don’t want AI to be defectively implemented is healthcare.
“Mitra Robot” was programmed to welcome the Prime Minister of India, Shri Narendra Modi, and Ivanka Trump using their names when they pressed on their country’s respective flags at a meeting. It so happened that both individuals pressed the buttons simultaneously leaving the robot confused and nonfunctional. A case of poor analysis and coding.
Unfortunately, this is by no means an unusual occurrence. Alexa made news in 2017 after it started ordering people dollhouses after hearing its name on TV. Having its algorithm tested in more realistic scenarios should have been, in hindsight, taken into consideration.
Have We Thoroughly Considered Contextual Factors and Human Behavioural Response?
When AI researchers from TU Dortmund, TU Munich and Ghent University of Belgium ran machine learning models to predict the winner of World Cup 2018, most got it totally wrong. Factors such as FIFA rankings, each country’s population, and GDP, bookmaker odds, how many players are playing in the same club, the average age, and how many Champions Leagues they have won were considered. None of these factors, however, account for the hard-to-quantify variables that play a great role in performance: motivation, culture and work behavior.
Microsoft’s chatbot “Tay” is a strong example of how neglecting basic human impulses can be harmful in AI implementation. Tay was a great learner. Unfortunately, many people interacted with it using crude, vulgar and racist remarks. It didn’t take long until he started applying the knowledge people shared with him.
The implications of failure to accurately assess the risks of AI implementation in human’s routine can be even graver. The case of the pedestrian in the US who was killed by an Uber test vehicle operating in self-drive mode rose what is to this date one of the biggest ethical debates on AI.
Final Remarks
If the answer to the three questions above is not a resounding “yes”, troubles may follow.
There are, of course, many other points that need to be considered in order to ensure any AI-related project is adequately implemented. The rush to stay ahead of the technology curve and insufficient data diversity are common culprits.
For organizations that fail to consider such basic premises as the ones listed, successful AI adoption is sure to remain nothing but an endless source of headaches. The reward for those learning how to implement AI that results in individual and social good can, of course, be high. Privilege, however, entails responsibility – noblesse oblige.
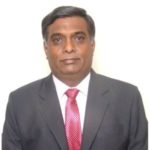
About the Author
Security Specialty Trainer; AWS Architect; CCC Master Trainer, Author of CTA, CTA+ & IoT; and Accredited DASA DevOps Trainer with 25+ years of IT experience.
Never miss an interesting article
Get our latest news, tutorials, guides, tips & deals delivered to your inbox.
Keep learning
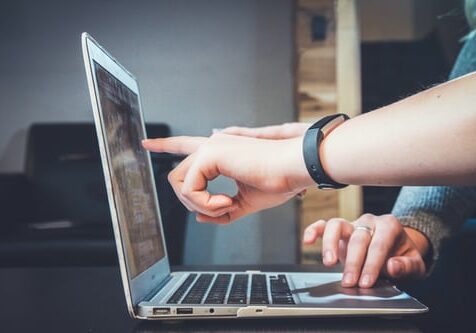